Lernkarten Bibliothek Aktivierungscode eingeben
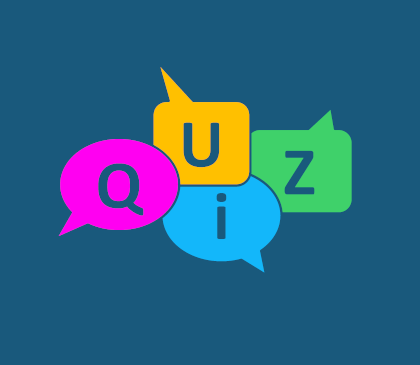
Verlosung: 50 x 1 PREMIUM-Abo
Beantworte 3 wichtige Fragen und gewinne 1 von 50 Card2Brain PREMIUM-Abos mit allen Zusatzfunktionen für noch mehr Lernerfolg in Schule, Studium und Beruf.
Spanisch
Spanisch
A2 (6)
Psychologie
Evidenzbasierte psychotherapeutische Verfahren I
Uni Würzburg, Master Psychotherapie
Französisch
Passé Composé
Mit être und avoir
Metalltechnik
HFP Metallbau Fachgespräch
Rechnungswesen 2 Betriebsbuchhaltung (BEBU)
Metalltechnik
HFP Metallbau Fachgespräch
Rechnungswesen 1 Betriebsbuchhaltung (FIBU)
Metalltechnik
HFP Metallbau Fachgespräch
Betriebsleitung 2
Metalltechnik
HFP Metallbau Fachgespräch
HFP Metallbui Personalführung 2